Similarly, manufacturing experts have been predicting for years that machines and robots, simply enabled by artificial intelligence (AI) and machine learning (ML), would lead to fully automated factories with no requirement of any human interaction. But even today’s most advanced self-learning AI systems cannot come close to matching the wide array of tasks a human engineer executes and require professionals to teach AI systems how to learn.
Why has AI failed to live up to the hype? In short, it is because people, both so-called experts and the public, have outsized expectations about the potential capabilities of AI. With all the buzz about AI and robots replacing humans, people assume that AI and ML can learn to reason and think abstractly and respond to unforeseen events the way humans do. That is not even close to the truth. In fact, the technological state of AI has the reasoning skills of a 4-year-old child – and therefore clearly unable to independently manage the complex operations that go on inside a factory. That is not to say that AI has nothing to offer. The current state of AI is capable of addressing many of manufacturing’s much-needed tasks, such as detecting patterns in quality failures and identifying potential efficiencies. But AI cannot respond to unknown environments, such as an unknown processing technique or an entirely new product to manufacture or figure out how to react to random and out of the ordinary situations, such as a new and previously unknown malfunction or a power outage.
HOW DOES MANUFACTURING BENEFIT FROM AI?
To unlock the full value of AI, there are two key prerequisites: Present a clear problem that can be solved with an objective truth and amass a large amount of training data – either real-world data or a problem that can be simulated. In short, let AI handle discreet, narrowly focused tasks and let humans handle complex problems with unknown variables. For example, AI is good at optimizing process efficiency in clearly defined process flow, while humans are much better at redesigning and optimizing shopfloor layouts. Similarly, AI is very strong at identifying quality issues in real time, but humans are still superior when it comes to resolving the root causes of these issues.
PROVEN USE CASES
Yet, there are many areas in manufacturing where AI is successfully being deployed today. In fact, more than 50 percent of large global companies will apply AI-enabled solutions in their supply-chain operations on a broad scale within the next five years! Consequently, worldwide spending is growing at staggering rates of more than 50 percent annually on cognitive and artificial intelligence systems and will surpass $20 billion in 2019. While there is a wide range of use cases being implemented in the supply chain, there are three especially promising use-case examples: Demand forecasting, production planning and scheduling, and visual pattern recognition. (See Exhibit 1.)
Exhibit 1: Three promising use-cases where AI is already successfully used today
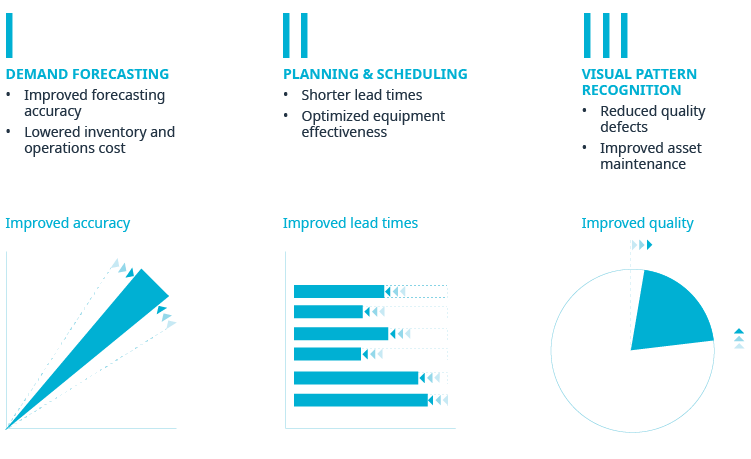
Source: Oliver Wyman analysis
1. Demand forecasting
One of the most challenging aspects of managing a supply chain is predicting the future demand for production. Machine learning is proving to be very effective at correlating hundreds and thousands of factors and deriving results that are much more accurate than can be achieved with traditional statistical methods. As a result, businesses can lower their inventory and operational costs and respond much quicker to customer demands. For example, by using a machine-learning engine in its supply chain, a leading provider of climate control products increased sales by 50 percent and inventory turnover by 25 percent. However, businesses should be aware that AI-based forecasting has its limitations and can only predict future outcomes that are directly correlated to previous events.
2. Production planning and scheduling
Machine learning can consider multiple constraints and optimize more effectively for them than traditional enterprise resource planning and production planning solutions. For instance, AI engines can digest machine data (speed, vibrations), environmental data (temperature, humidity), planning parameters (waiting times), forecasting data (demand), and more. They then correlate these vast amounts of input data to the output value of key metrics. By uncovering patterns in positive outcomes, they can derive insights for improving business performance. In addition, shop floor operations can be cross-linked with AI- enabled scheduling in real time to improve decision-making at each production run in a feedback loop. As a result, businesses are reducing lead times for components and parts, improving equipment utilization by up to 85 percent.
3. Visual pattern recognition
AI is also proving to be very effective at automating visual quality inspection in factories and throughout logistics hubs isolating damaged products and wear. Modern AI systems can be trained to recognize complex visual patterns using deep neural networks that are able to identify even microscopically small material defects. For example, one of the world’s largest logistics companies leverages a powerful AI engine to determine if a shipping container was damaged and to recommend the best course of action to repair the assets in real time. Similarly, precision machinery providers can guarantee 100 percent defect-free products by applying fully automated vision inspection systems. These examples show: while the fully automated, “zero-human“ factory remains more of a science-fiction dream than a reality, there are already many tangible use cases – especially in supply chain – that are boosting business performance today.